Data analysis: Diode full-wave rectification¶
Load packages¶
import matplotlib
import matplotlib.pyplot as plt # importar a bilioteca pyplot para fazer gráficos
from matplotlib import colors
#Comandos opcionais para formatar gráfico
font = {'family' : 'Arial',
'weight' : 'normal',
'size' : 12}
lines = {'linewidth' : 3.0}
figure = {'figsize' : [6.0, 6/1.6]}
matplotlib.rc('font', **font)
matplotlib.rc('lines', **lines)
matplotlib.rc('figure', **figure)
import numpy as np # importar a biblioteca Numpy para lidar com matrizes
import pandas as pd # importa bilioteca pandas para lidar com processamento de dados
import os #com
import glob
from scipy.optimize import curve_fit # pacote para ajuste de curvas
# navegar pelas pastas
Getting the list of files¶
If you want to find out in which folder your located at the moment, use the command os.getcwd()
:
os.getcwd() # aonde estou?
'/Users/gsw/GitHub/F540_jbook/guides/exp2/dados'
This is important because it may affect how you load data in the cell below:
The command
path = os.getcwd()
will assign to the variablepath
the current folderIf your data files are within this folder, then you should be able to load them right away
#Colocando nomes das pastas e arquivos
path = os.getcwd()
print(path)
/Users/gsw/GitHub/F540_jbook/guides/exp2/dados
glob.glob('*')
will list all files in current folder, including subfolders:
glob.glob('*')
['dados_onda_completa',
'dados_meia_onda.zip',
'dados_onda_completa.zip',
'analise_meia_onda_teoria_fig.png',
'001_meia_onda_c_R_330.dat',
'analisa_dados_diodo.ipynb']
I know my data is within the
dados_onda_completa
folder, so I will list the files within it.Im Unix OS (Mac and Linux), I will provide the string
dados_onda_completa/*
. But in Windows, it will need to bedados_onda_completa\*
.To make sure this command will work regardless of you OS, I will explore the command
os.path.join
, which will determine automatically whether/
or\
is to be used:
os.path.join('dados_onda_completa','*')
'dados_onda_completa/*'
file_list = sorted(glob.glob(os.path.join('dados_onda_completa','*')))
print(file_list)
['dados_onda_completa/013_onda_completa_R_330.dat', 'dados_onda_completa/013_onda_completa_R_330_fig.png', 'dados_onda_completa/014_onda_completa_C_R_330.dat', 'dados_onda_completa/014_onda_completa_C_R_330_fig.png', 'dados_onda_completa/015_onda_completa_C_R_352.dat', 'dados_onda_completa/015_onda_completa_C_R_352_fig.png', 'dados_onda_completa/016_onda_completa_C_R_412.dat', 'dados_onda_completa/016_onda_completa_C_R_412_fig.png', 'dados_onda_completa/017_onda_completa_C_R_510.dat', 'dados_onda_completa/017_onda_completa_C_R_510_fig.png', 'dados_onda_completa/018_onda_completa_C_R_610.dat', 'dados_onda_completa/018_onda_completa_C_R_610_fig.png', 'dados_onda_completa/019_onda_completa_C_R_820.dat', 'dados_onda_completa/019_onda_completa_C_R_820_fig.png', 'dados_onda_completa/020_onda_completa_C_R_1000.dat', 'dados_onda_completa/020_onda_completa_C_R_1000_fig.png', 'dados_onda_completa/021_onda_completa_C_R_1200.dat', 'dados_onda_completa/021_onda_completa_C_R_1200_fig.png', 'dados_onda_completa/022_onda_completa_C_R_2700.dat', 'dados_onda_completa/022_onda_completa_C_R_2700_fig.png', 'dados_onda_completa/023_onda_completa_C_R_4700.dat', 'dados_onda_completa/023_onda_completa_C_R_4700_fig.png', 'dados_onda_completa/024_onda_completa_C_R_9100.dat', 'dados_onda_completa/024_onda_completa_C_R_9100_fig.png', 'dados_onda_completa/025_onda_completa_C_R_aberto.dat', 'dados_onda_completa/025_onda_completa_C_R_aberto_fig.png']
Note usage of the python fucntion
sorted()
to sort the files in increasing name order.The list above is fine and we can access its content using integer indexes (starting at zero):
file_list[0]
'dados_onda_completa/013_onda_completa_R_330.dat'
Instead, I will use the command
pd.Series(file_list)
from the Pandas package, this will show the file names with the corresponding indexes:
pd.Series(file_list)
0 dados_onda_completa/013_onda_completa_R_330.dat
1 dados_onda_completa/013_onda_completa_R_330_fi...
2 dados_onda_completa/014_onda_completa_C_R_330.dat
3 dados_onda_completa/014_onda_completa_C_R_330_...
4 dados_onda_completa/015_onda_completa_C_R_352.dat
5 dados_onda_completa/015_onda_completa_C_R_352_...
6 dados_onda_completa/016_onda_completa_C_R_412.dat
7 dados_onda_completa/016_onda_completa_C_R_412_...
8 dados_onda_completa/017_onda_completa_C_R_510.dat
9 dados_onda_completa/017_onda_completa_C_R_510_...
10 dados_onda_completa/018_onda_completa_C_R_610.dat
11 dados_onda_completa/018_onda_completa_C_R_610_...
12 dados_onda_completa/019_onda_completa_C_R_820.dat
13 dados_onda_completa/019_onda_completa_C_R_820_...
14 dados_onda_completa/020_onda_completa_C_R_1000...
15 dados_onda_completa/020_onda_completa_C_R_1000...
16 dados_onda_completa/021_onda_completa_C_R_1200...
17 dados_onda_completa/021_onda_completa_C_R_1200...
18 dados_onda_completa/022_onda_completa_C_R_2700...
19 dados_onda_completa/022_onda_completa_C_R_2700...
20 dados_onda_completa/023_onda_completa_C_R_4700...
21 dados_onda_completa/023_onda_completa_C_R_4700...
22 dados_onda_completa/024_onda_completa_C_R_9100...
23 dados_onda_completa/024_onda_completa_C_R_9100...
24 dados_onda_completa/025_onda_completa_C_R_aber...
25 dados_onda_completa/025_onda_completa_C_R_aber...
dtype: object
Another way of achieving a similar result is using a for loop:
[print([i, file]) for i,file in enumerate(file_list)];
[0, 'dados_onda_completa/013_onda_completa_R_330.dat']
[1, 'dados_onda_completa/013_onda_completa_R_330_fig.png']
[2, 'dados_onda_completa/014_onda_completa_C_R_330.dat']
[3, 'dados_onda_completa/014_onda_completa_C_R_330_fig.png']
[4, 'dados_onda_completa/015_onda_completa_C_R_352.dat']
[5, 'dados_onda_completa/015_onda_completa_C_R_352_fig.png']
[6, 'dados_onda_completa/016_onda_completa_C_R_412.dat']
[7, 'dados_onda_completa/016_onda_completa_C_R_412_fig.png']
[8, 'dados_onda_completa/017_onda_completa_C_R_510.dat']
[9, 'dados_onda_completa/017_onda_completa_C_R_510_fig.png']
[10, 'dados_onda_completa/018_onda_completa_C_R_610.dat']
[11, 'dados_onda_completa/018_onda_completa_C_R_610_fig.png']
[12, 'dados_onda_completa/019_onda_completa_C_R_820.dat']
[13, 'dados_onda_completa/019_onda_completa_C_R_820_fig.png']
[14, 'dados_onda_completa/020_onda_completa_C_R_1000.dat']
[15, 'dados_onda_completa/020_onda_completa_C_R_1000_fig.png']
[16, 'dados_onda_completa/021_onda_completa_C_R_1200.dat']
[17, 'dados_onda_completa/021_onda_completa_C_R_1200_fig.png']
[18, 'dados_onda_completa/022_onda_completa_C_R_2700.dat']
[19, 'dados_onda_completa/022_onda_completa_C_R_2700_fig.png']
[20, 'dados_onda_completa/023_onda_completa_C_R_4700.dat']
[21, 'dados_onda_completa/023_onda_completa_C_R_4700_fig.png']
[22, 'dados_onda_completa/024_onda_completa_C_R_9100.dat']
[23, 'dados_onda_completa/024_onda_completa_C_R_9100_fig.png']
[24, 'dados_onda_completa/025_onda_completa_C_R_aberto.dat']
[25, 'dados_onda_completa/025_onda_completa_C_R_aberto_fig.png']
Reading the files¶
reading a single file¶
Note the use of the sep='\t'
argument for the pd.read_csv
command. It was used because the data files used a TAB character for separating values.
The output file will be a Pandas DataFrame object:
file = file_list[0]
print(file)
df = pd.read_csv(file,sep='\t') # DataFrame segundo Pandasdf
df.columns = ['tempo(s)','ch1(V)','ch2(V)']
df.head() # preview the first few rows
dados_onda_completa/013_onda_completa_R_330.dat
tempo(s) | ch1(V) | ch2(V) | |
---|---|---|---|
0 | 0.00002 | 1.2 | 0.6 |
1 | 0.00004 | 1.4 | 0.6 |
2 | 0.00006 | 1.4 | 0.8 |
3 | 0.00008 | 1.6 | 1.0 |
4 | 0.00010 | 1.8 | 1.0 |
We can access columns using either of the two approaches:
Using the column name, for example,
df['ch1(V)']
will give us column'ch1(V)'
contentUsing indexes:
df.iloc[:,0]
- All rows of columntempo(s)
df.iloc[0,0]
- first row of columntempo(s)
df.iloc[:,1]
- All rows of columnch1(V)
df.iloc[:,2]
- All rows of columnch2(V)
For example:
df.iloc[:,0]
0 0.00002
1 0.00004
2 0.00006
3 0.00008
4 0.00010
...
2495 0.04992
2496 0.04994
2497 0.04996
2498 0.04998
2499 0.05000
Name: tempo(s), Length: 2500, dtype: float64
Below use Numpy functions to calculate:
mean value
max and min voltages Note that Numpy functions works seamlessly with Pandas DataFrames:
V1med = np.mean(df['ch1(V)'])
V1max = np.max(df['ch1(V)'])
V1min = np.min(df['ch1(V)'])
print('tensões=',[V1med,V1max,V1min])
#---
V2med = np.mean(df['ch2(V)'])
V2max = np.max(df['ch2(V)'])
V2min = np.min(df['ch2(V)'])
print('tensões=',[V2med,V2max,V2min])
tensões= [0.09839999999999946, 15.8, -15.6]
tensões= [9.34344, 14.8, -0.2]
Plotting the time waveforms using Matplotlib¶
#----
fig,ax = plt.subplots(1,2,figsize=(10,3), sharey=True)
#------------------------
ax0=ax[0]
#--
ax0.plot(1e3*df['tempo(s)'],df['ch1(V)'])
ax0.axhline(V1med,color='green',linestyle='--',label='$V_{dc}$'+'={:3.2f} V'.format(V1med))
ax0.axhline(V1max,color='red',linestyle='--',label='$V_{max}$'+'={:3.2f} V'.format(V1max))
ax0.axhline(V1min,color='blue',linestyle='--',label='$V_{min}$'+'={:3.2f} V'.format(V1min))
#--
ax0.grid(True)
ax0.set_xlabel('Tempo (ms)')
ax0.set_ylabel('Tensão (V)')
ax0.legend(loc='best')
#------------------------
ax0=ax[1]
ax0.plot(1e3*df['tempo(s)'],df['ch2(V)'])
#--
ax0.axhline(V2med,color='green',linestyle='--',label='$V_{dc}$'+'={:3.2f} V'.format(V2med))
ax0.axhline(V2max,color='red',linestyle='--',label='$V_{max}$'+'={:3.2f} V'.format(V2max))
ax0.axhline(V2min,color='blue',linestyle='--',label='$V_{min}$'+'={:3.2f} V'.format(V2min))
#--
ax0.grid(True)
ax0.set_xlabel('Tempo (ms)')
#ax0.set_ylabel('Tensão (V)')
ax0.legend(loc='best')
#------------------
plt.tight_layout()
#----
st = fig.suptitle('dado: '+file)
# shift subplots down:
st.set_y(1.02)
#---
#plt.savefig(file[0:-4]+'_fig.pdf')
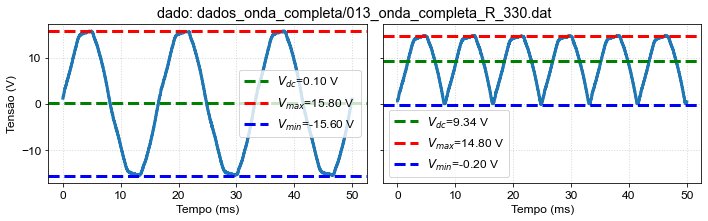
Loading and plotting multiple files at once¶
from myst_nb import glue
glue("file_print",pd.Series(file_list))
0 dados_onda_completa/013_onda_completa_R_330.dat
1 dados_onda_completa/013_onda_completa_R_330_fi...
2 dados_onda_completa/014_onda_completa_C_R_330.dat
3 dados_onda_completa/014_onda_completa_C_R_330_...
4 dados_onda_completa/015_onda_completa_C_R_352.dat
5 dados_onda_completa/015_onda_completa_C_R_352_...
6 dados_onda_completa/016_onda_completa_C_R_412.dat
7 dados_onda_completa/016_onda_completa_C_R_412_...
8 dados_onda_completa/017_onda_completa_C_R_510.dat
9 dados_onda_completa/017_onda_completa_C_R_510_...
10 dados_onda_completa/018_onda_completa_C_R_610.dat
11 dados_onda_completa/018_onda_completa_C_R_610_...
12 dados_onda_completa/019_onda_completa_C_R_820.dat
13 dados_onda_completa/019_onda_completa_C_R_820_...
14 dados_onda_completa/020_onda_completa_C_R_1000...
15 dados_onda_completa/020_onda_completa_C_R_1000...
16 dados_onda_completa/021_onda_completa_C_R_1200...
17 dados_onda_completa/021_onda_completa_C_R_1200...
18 dados_onda_completa/022_onda_completa_C_R_2700...
19 dados_onda_completa/022_onda_completa_C_R_2700...
20 dados_onda_completa/023_onda_completa_C_R_4700...
21 dados_onda_completa/023_onda_completa_C_R_4700...
22 dados_onda_completa/024_onda_completa_C_R_9100...
23 dados_onda_completa/024_onda_completa_C_R_9100...
24 dados_onda_completa/025_onda_completa_C_R_aber...
25 dados_onda_completa/025_onda_completa_C_R_aber...
dtype: object
Now that we know how to handle a single file, we can use a for
loop to sequentially open and process all files. For example, I manually extracted from the file names the values of resistance associated with each file:
res_val = np.array([327,327,349,404,505,603,820,993,1185,2640,4640,8880,1e8])
#----------------
#mapa de cores
cm=plt.get_cmap('viridis')
norm = colors.Normalize(vmin = 330,vmax = 1e4)
#----------------
#initialize python lists to store the relevant quantities
vmax_vec = [] # max voltage
vmed_vec = [] # mean value
vrip_vec = [] # ripple voltage
label_vec = [] # label
#---
fig,ax = plt.subplots(1,2,figsize=(10,5), sharey=True)
for ii,file in enumerate(file_list):#[1:-3]
#----
df = pd.read_csv(file,sep='\t') # DataFrame segundo Pandasdf
df.columns = ['tempo(s)','ch1(V)','ch2(V)']
#----
V1med = np.mean(df['ch1(V)'])
V1max = np.max(df['ch1(V)'])
V1min = np.min(df['ch1(V)'])
#---
V2med = np.mean(df['ch2(V)'])
V2max = np.max(df['ch2(V)'])
V2min = np.min(df['ch2(V)'])
#--
vmax_vec.append(V2max)
vmed_vec.append(V2med)
vrip_vec.append(V2max-V2min)
#----
#------------------------
#grafica apenas o primeiro
if ii==0:
ax0=ax[0]
#--
ax0.plot(1e3*df['tempo(s)'],df['ch1(V)'])
ax0.axhline(V1med,color='green',linestyle='--',label='$V_{dc}$'+'={:3.2f} V'.format(V1med))
ax0.axhline(V1max,color='red',linestyle='--',label='$V_{max}$'+'={:3.2f} V'.format(V1max))
ax0.axhline(V1min,color='blue',linestyle='--',label='$V_{min}$'+'={:3.2f} V'.format(V1min))
#--
ax0.grid(True)
ax0.set_xlabel('Tempo (ms)')
ax0.set_ylabel('Tensão (V)')
ax0.legend(loc='best')
#------------------------
ax0=ax[1]
if ii==0: # different label in this case
label_vec.append('sem cap.')
ax0.plot(1e3*df['tempo(s)'],df['ch2(V)'], lw=1,ls='--',
color='k',
label=label_vec[ii])
elif ii==len(res_val)-1: # different label in this case
label_vec.append('aberto (sem res.)')
ax0.plot(1e3*df['tempo(s)'],df['ch2(V)'], lw=1,ls='--',
color=cm((norm(res_val[ii])**(0.1))),
label=label_vec[ii])
else: # common labels
label_vec.append('{:}'.format(res_val[ii]))
ax0.plot(1e3*df['tempo(s)'],df['ch2(V)'], lw=1,
color=cm((norm(res_val[ii])**(0.1))),
label=label_vec[ii])
#--
ax0.grid(True)
ax0.set_xlabel('Tempo (ms)')
#ax0.set_ylabel('Tensão (V)')
ax0.legend(loc='center left',bbox_to_anchor=(1,0.5))
#------------------
plt.tight_layout()
#----
st = fig.suptitle('dado: meia onda ')
# shift subplots down:
st.set_y(1.02)
#---
# plt.savefig('todos_dados_meia_onda'+'_fig.pdf')
#plt.savefig('todos_dados_onda_completa'+'_fig.pdf')
---------------------------------------------------------------------------
UnicodeDecodeError Traceback (most recent call last)
<ipython-input-15-de959cbe8f3f> in <module>
14 for ii,file in enumerate(file_list):#[1:-3]
15 #----
---> 16 df = pd.read_csv(file,sep='\t') # DataFrame segundo Pandasdf
17 df.columns = ['tempo(s)','ch1(V)','ch2(V)']
18 #----
/Library/Frameworks/Python.framework/Versions/3.7/lib/python3.7/site-packages/pandas/io/parsers.py in read_csv(filepath_or_buffer, sep, delimiter, header, names, index_col, usecols, squeeze, prefix, mangle_dupe_cols, dtype, engine, converters, true_values, false_values, skipinitialspace, skiprows, skipfooter, nrows, na_values, keep_default_na, na_filter, verbose, skip_blank_lines, parse_dates, infer_datetime_format, keep_date_col, date_parser, dayfirst, cache_dates, iterator, chunksize, compression, thousands, decimal, lineterminator, quotechar, quoting, doublequote, escapechar, comment, encoding, dialect, error_bad_lines, warn_bad_lines, delim_whitespace, low_memory, memory_map, float_precision)
684 )
685
--> 686 return _read(filepath_or_buffer, kwds)
687
688
/Library/Frameworks/Python.framework/Versions/3.7/lib/python3.7/site-packages/pandas/io/parsers.py in _read(filepath_or_buffer, kwds)
450
451 # Create the parser.
--> 452 parser = TextFileReader(fp_or_buf, **kwds)
453
454 if chunksize or iterator:
/Library/Frameworks/Python.framework/Versions/3.7/lib/python3.7/site-packages/pandas/io/parsers.py in __init__(self, f, engine, **kwds)
944 self.options["has_index_names"] = kwds["has_index_names"]
945
--> 946 self._make_engine(self.engine)
947
948 def close(self):
/Library/Frameworks/Python.framework/Versions/3.7/lib/python3.7/site-packages/pandas/io/parsers.py in _make_engine(self, engine)
1176 def _make_engine(self, engine="c"):
1177 if engine == "c":
-> 1178 self._engine = CParserWrapper(self.f, **self.options)
1179 else:
1180 if engine == "python":
/Library/Frameworks/Python.framework/Versions/3.7/lib/python3.7/site-packages/pandas/io/parsers.py in __init__(self, src, **kwds)
2006 kwds["usecols"] = self.usecols
2007
-> 2008 self._reader = parsers.TextReader(src, **kwds)
2009 self.unnamed_cols = self._reader.unnamed_cols
2010
pandas/_libs/parsers.pyx in pandas._libs.parsers.TextReader.__cinit__()
pandas/_libs/parsers.pyx in pandas._libs.parsers.TextReader._get_header()
UnicodeDecodeError: 'utf-8' codec can't decode byte 0x89 in position 0: invalid start byte
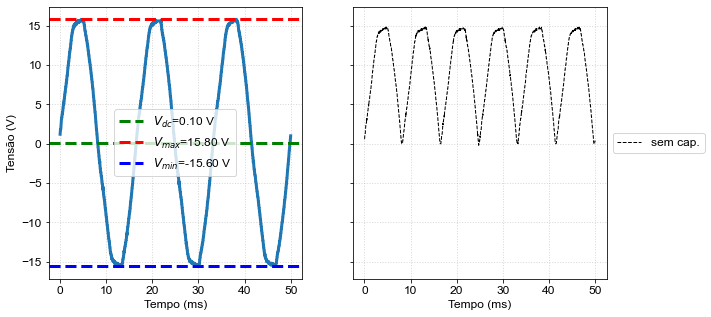
Characteristics of the diode-rectified voltage source¶
Below we explore the function curve_fit
from the Scipy package (loaded at the beggining of this file with from scipy.optimize import curve_fit
Fitting model to data¶
Rinverse = 1e3/res_val # inverse of resistance in mS ([S]=1/[Ohm])
T=1/60 # period
#------------------------------------
#define fittting function
#theory
vmax=np.mean(vmax_vec) # we assume the input voltagevmax_vec is approximately constant during the experiment, verify!!
def vripple(r,c0):
return vmax_vec[0]*(1-np.exp(-T/(r*c0)) )
pfit, pcov = curve_fit(vripple, res_val,vrip_vec, p0=22e-6)
c0=pfit[0]
print('fitted capacitance, c=',c0)
fitted capacitance, c= 5.475089763087214e-05
Comparing data and fitted model¶
#------------------------------------
#Generate the "theory" curves from our fitted model
vripT1_vec= (vmax_vec[0])/(c0*res_val)*T #linearizado
vripT2_vec= vmax_vec[0]*(1 - np.exp(-T/(res_val*c0)) ) #completo
#------------------------------------
fig,ax = plt.subplots(2,1,figsize=(5,5), sharex=True)
ax0=ax[0]
ax0.grid(True)
ax0.set_ylabel('Tensão média $V_{dc}$ (V)')
#------------------------------------
for ii,r0 in enumerate(res_val):
# this if is to skip the case with no capacitor (ii=0) and no resistor (ii=12)
if (ii>=1 and ii<len(res_val)-1):
ax[0].scatter(Rinverse[ii],vmed_vec[ii],
color=cm((norm(res_val[ii])**(1/8))),
label=label_vec[ii])
ax[1].scatter(Rinverse[ii],vrip_vec[ii],
color=cm((norm(res_val[ii])**(1/8))),
label=label_vec[ii])
#---------------
ax0=ax[1]
ax0.grid(True)
#-------------------
ax0.plot(Rinverse,vripT1_vec,'-',label ='linear')
ax0.plot(Rinverse,vripT2_vec,'-',label = 'completo')
ax0.set_ylabel('Tensão de Ripple $V_{rip}$ (V)')
ax0.set_xlabel('$R^{-1}$ (mS)')
handles, labels = ax0.get_legend_handles_labels()
ax0.legend(loc='center left',bbox_to_anchor=(1,1))
#----
st = fig.suptitle('dado: simulação meia onda c=22 μF, cfit={:2.1f} μF'.format(1e6*c0))
# shift subplots down:
st.set_y(1.02)
plt.tight_layout()
plt.subplots_adjust(hspace=0.1)
#plt.savefig('analise_meia_onda_teoria'+'_fig.png', bbox_inches='tight')
/Users/gsw/Library/Python/3.7/lib/python/site-packages/ipykernel_launcher.py:15: RuntimeWarning: invalid value encountered in double_scalars
from ipykernel import kernelapp as app
/Users/gsw/Library/Python/3.7/lib/python/site-packages/ipykernel_launcher.py:18: RuntimeWarning: invalid value encountered in double_scalars
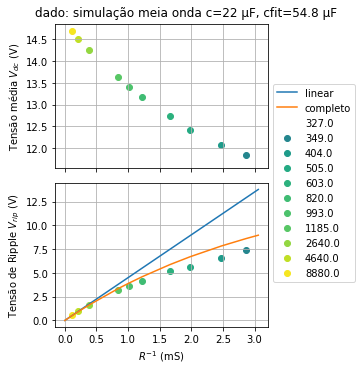
Generating time traces for each data¶
Let’s say you are not sure about the what the min/max/mean script above did and want to visually inspect the result, you should always do this, never trust automation without double-checking the results.
Below we show an example of how could you generate a time-trace plot for each experimental curve and make sure your script is doing the right thing.
It will save multiple files in the current folder
plt.savefig(file[0:-4]+'_fig.png')
, each containing the max/min/mean identification for each dataset
for file in file_list:
#----
df = pd.read_csv(file,sep='\t') # DataFrame segundo Pandasdf
df.columns = ['tempo(s)','ch1(V)','ch2(V)']
#----
V1med = np.mean(df['ch1(V)'])
V1max = np.max(df['ch1(V)'])
V1min = np.min(df['ch1(V)'])
print('tensões=',[V1med,V1max,V1min])
#---
V2med = np.mean(df['ch2(V)'])
V2max = np.max(df['ch2(V)'])
V2min = np.min(df['ch2(V)'])
print('tensões=',[V2med,V2max,V2min])
#----
fig,ax = plt.subplots(1,2,figsize=(10,3), sharey=True)
#------------------------
ax0=ax[0]
#--
ax0.plot(1e3*df['tempo(s)'],df['ch1(V)'])
ax0.axhline(V1med,color='green',linestyle='--',label='$V_{dc}$'+'={:3.2f} V'.format(V1med))
ax0.axhline(V1max,color='red',linestyle='--',label='$V_{max}$'+'={:3.2f} V'.format(V1max))
ax0.axhline(V1min,color='blue',linestyle='--',label='$V_{min}$'+'={:3.2f} V'.format(V1min))
#--
ax0.grid(True)
ax0.set_xlabel('Tempo (ms)')
ax0.set_ylabel('Tensão (V)')
ax0.legend(loc='best')
#------------------------
ax0=ax[1]
ax0.plot(1e3*df['tempo(s)'],df['ch2(V)'])
#--
ax0.axhline(V2med,color='green',linestyle='--',label='$V_{dc}$'+'={:3.2f} V'.format(V2med))
ax0.axhline(V2max,color='red',linestyle='--',label='$V_{max}$'+'={:3.2f} V'.format(V2max))
ax0.axhline(V2min,color='blue',linestyle='--',label='$V_{min}$'+'={:3.2f} V'.format(V2min))
#--
ax0.grid(True)
ax0.set_xlabel('Tempo (ms)')
#ax0.set_ylabel('Tensão (V)')
ax0.legend(loc='best')
#------------------
plt.tight_layout()
#----
st = fig.suptitle('dado: '+file)
# shift subplots down:
st.set_y(1.02)
#---
plt.savefig(file[0:-4]+'_fig.png')
plt.close()
tensões= [0.09839999999999946, 15.8, -15.6]
tensões= [9.34344, 14.8, -0.2]
tensões= [0.09295999999999985, 15.8, -15.6]
tensões= [11.68384, 14.8, 7.4]
tensões= [0.09519999999999963, 15.8, -15.6]
tensões= [11.8356, 15.0, 7.6]
tensões= [0.09335999999999949, 15.8, -15.6]
tensões= [12.064160000000001, 14.8, 8.200000000000001]
tensões= [0.09704000000000014, 15.8, -15.6]
tensões= [12.42704, 14.8, 9.2]
tensões= [0.10192000000000007, 15.8, -15.6]
tensões= [12.732, 15.0, 9.8]
tensões= [0.10503999999999979, 15.8, -15.6]
tensões= [13.176, 15.0, 10.8]
tensões= [0.10567999999999957, 15.8, -15.6]
tensões= [13.4032, 15.0, 11.4]
tensões= [0.10536000000000022, 16.0, -15.8]
tensões= [13.627120000000001, 15.0, 11.8]
tensões= [0.10728000000000029, 15.8, -15.6]
tensões= [14.259520000000002, 15.0, 13.4]
tensões= [0.10799999999999964, 15.8, -15.6]
tensões= [14.5064, 15.0, 14.0]
tensões= [0.1056, 15.8, -15.6]
tensões= [14.691679999999998, 15.0, 14.4]
tensões= [0.10863999999999978, 16.0, -15.6]
tensões= [15.179039999999999, 15.2, 15.0]